Chin-Fu Tsang Coupled Processes Award Lecture
Predicting Transport, Mixing, and Reaction in Fractured Porous Media
Peter K. Kang
Department of Earth and Environmental Sciences, University of Minnesota, Twin Cities
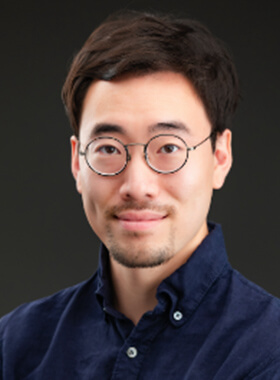
Biosketch
I am a geoscientist whose research focuses on the physics of flow and reactive transport in porous and fractured media. My research group combines high-performance numerical simulations, visual laboratory experiments, stochastic upscaling, and machine learning to elucidate how the coupling between multiple processes controls transport processes in fractured porous media (https://pkkang.com).
I joined the Department of Earth and Environmental Science at the University of Minnesota as an Assistant Professor and a Gibson Chair of Hydrogeology in 2018. I was a researcher at Korea Institute of Science and Technology (KIST) from 2015-2018, and was a postdoctoral associate in the Earth Resources Laboratory (ERL) at MIT before joining KIST. I received my MSc (2010) and PhD (2014) in Civil & Environmental Engineering at MIT, and obtained BSc of Civil, Urban & Geosystem engineering at Seoul National University in South Korea with summa cum laude in 2008.
Introduction of the Lecture
Fluid flow and reactive transport in geologic fractures control many critical natural and engineered processes in the subsurface. For example, 99% of global unfrozen freshwater is stored in groundwater systems, and groundwater flow is often dominated by fracture flows. Also, engineered carbon mineralization is considered a key solution for climate change, and fractures serve as highways for the delivery of CO2 into mafic and ultramafic rocks, determining the efficiency of carbon mineralization. However, predicting transport processes in fractured porous media is challenging due to the multi-scale heterogeneity inherent to subsurface systems and the strong coupling between processes (i.e., coupled thermo-hydro-mechanical-biological-chemical processes).
In this talk, I will present how my research group uses cutting-edge methods to advance our fundamental understanding of coupled processes as well as our capacity to predict transport processes in fractured porous media. In particular, I will highlight the role of fluid inertia and pore-scale flow structures on transport, mixing, and biogeochemical reactions and our efforts to upscale transport processes in fractured porous media. The talk will conclude by sharing our ongoing efforts to extend the research findings to fractured aquifer sites.