A Clearer Look at Induced Seismicity with Deep Learning
Gregory C. Beroza
Stanford University, USA
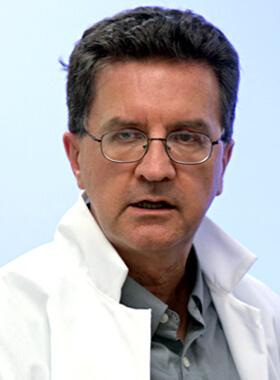
Biosketch
My research focus is on analyzing seismograms to understand how earthquakes work and to quantify the hazards they pose. My research interests include shallow earthquakes, intermediate-depth earthquakes, induced earthquakes, and slow earthquakes. We are working to improve earthquake monitoring in all settings by applying data mining and machine learning techniques to large continuous seismic waveform data. We also work on methods to anticipate the strength of shaking in earthquakes using the ambient seismic field. I currently serve as the Co-Director of both the Southern California Earthquake Center and the Stanford Center for Induced and Triggered Seismicity.
Introduction of the Lecture
Deep learning is having an impact across seismology, but its greatest impact has been in earthquake monitoring. The introduction of deep learning into earthquake monitoring workflows can reduce the detection threshold by one unit of magnitude or more, and because the number of earthquakes increases rapidly as magnitude decreases, detecting somewhat smaller earthquakes dramatically increases available information. The new generation of information-rich earthquake catalogs have yielded important insights into induced seismicity and hold the promise for yet more insights through the application of AI methods to the catalogs themselves.